Text-mining with the SimText toolset
Author(s) |
![]() ![]() |
Reviewers |
|
OverviewQuestions:
Objectives:
How can I automatically collect PubMed data for a set of biomedical entities such as genes?
How can I analyze similarities among biomedical entities based on PubMed data on large-scale?
Requirements:
Learn how to use the SimText toolset
Upload a table with biomedical entities in Galaxy
Retrieve PubMed data for each of the biomedical entities
Extract biomedical terms from the PubMed data for each biomedical entity
Analyze the similarity among the biomedical entities based on the extracted data in an interactive app
Time estimation: 1 hourSupporting Materials:Published: Apr 5, 2021Last modification: Mar 5, 2024License: Tutorial Content is licensed under Creative Commons Attribution 4.0 International License. The GTN Framework is licensed under MITpurl PURL: https://gxy.io/GTN:T00272version Revision: 7
Literature exploration in PubMed on a large number of biomedical entities (e.g., genes, diseases, or experiments) can be time-consuming and challenging, especially when assessing associations between entities. Here, we use SimText, a toolset for literature research that allows you to collect text from PubMed for any given set of biomedical entities, extract associated terms, and analyze similarities among them and their key characteristics in an interactive tool.
This tutorial is based on a proof-of-concept example given in Gramm et al. 2020. We are going to analyze similarities among 95 genes based on their associated biomedical terms in the literature, and compare their pre-existing disorder categories to their grouping based on the literature.
The workflow combines 3 main steps, starting with the retrieval of PubMed data for each of the genes. We then use the PubMed data from each gene to extract related scientific terms that are all combined in one large binary matrix. Finally, we explore the generated data in an interactive tool that performs different unsupervised machine-learning algorithms to analyze the similarities/ the grouping among the genes based on their extracted terms from the literature.
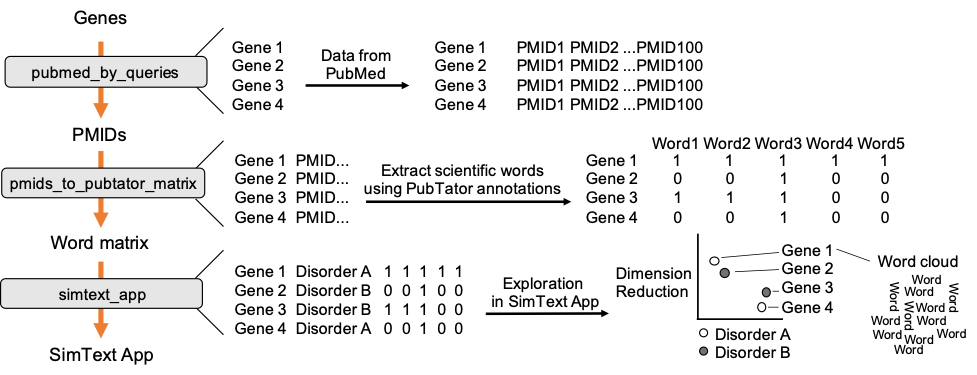
AgendaIn this tutorial, we will cover:
Input data
The input data is a simple table with the genes we want to analyze as well as their pre-existing grouping (the grouping is required later on to compare it to our text-based gene grouping). In order for the tools to recognize the column with the biomedical entities of interest, our 95 genes, the column name should start with “ID_”, and for the grouping variable with “GROUPING_”.
Hands On: Data upload
Create a new history for this tutorial
To create a new history simply click the new-history icon at the top of the history panel:
Import the input file from Zenodo
https://zenodo.org/records/4638516/files/clingen_data
- Copy the link location
Click galaxy-upload Upload Data at the top of the tool panel
- Select galaxy-wf-edit Paste/Fetch Data
Paste the link(s) into the text field
Press Start
- Close the window
Retrieval of PubMed data
In the first step we collect PubMed data for each of the genes. The genes are used as search queries to download a defined number of PMIDs, here up to 500, from PubMed. The PMIDs are saved in additional columns of our input data.
To speed up the the download of PubMed data users can obtain an API key from the settings page of their NCBI account (to create an account, visit http://www.ncbi.nlm.nih.gov/account/) and add it to the Galaxy user-preferences (User → Preferences → Manage Information).
Hands On: Step 1: PubMed query tool
- Run PubMed query ( Galaxy version 0.0.2) with the following parameters:
- param-file “Input file with query terms”: Input dataset
- “Number of PMIDs (or abstracts) to save per ID”:
500
CommentThe tool is also able to save the abstracts as text instead of their PMIDs. This feature is used for another type of analysis (see Gramm et al. 2020), or can be used if the user wants to use the tool independent from a workflow to retrieve many abstract texts at once. For the next step of our example in this tutorial, only PMIDs are required.
Extraction of biomedical terms from PubMed abstracts
Next, we extract the 100 most frequent ‘Disease’ and ‘Gene’ terms (PubTator annotations) from the PubMed data. All genes with their 100 associated terms are then combined in one large binary matrix. Each row represents a gene and each column one of the extracted terms. This matrix is later used to find similar genes, i.e. genes that have many common terms associated with them.
Hands On: Extraction of PubTator annotations
- Run PMIDs to PubTator ( Galaxy version 0.0.2) with the following parameters:
- param-file “Input file with PMID IDs”: output of PubMed query tool
- “categories”:
Genes Diseases
- “Number of most frequent terms/IDs to extract.”:
100
Comment: PubTatorPubTator annotates terms of the following categories: Gene, Disease, Mutation, Species and Chemical. In this example we chose to only extract gene and disease terms but you can also select other categories if you are interested in those.
Exploration of data in interactive tool
After generating the large binary matrix, we can explore the similarities/ the grouping among the genes in the interactive SimText tool. The following features are generated:
- Word clouds for each gene
- Dimension reduction and hierarchical clustering of the binary matrix
- Calculation of the adjusted rand index (similarity between text-based grouping and the pre-existing disorder categories)
- Table with terms and their frequency among the genes
Hands On: Explore data interactively
- Run interactive_tool_simtext_app with the following parameters:
- param-file “Input file”: initial input file with genes and pre-existing grouping
- param-file “Matrix file”: output of PMIDs to PubTator tool
Open interactive tool
- Go to User > Active InteractiveTools
- Wait for the SimText app to be running (Job Info)
- Click on SimText app
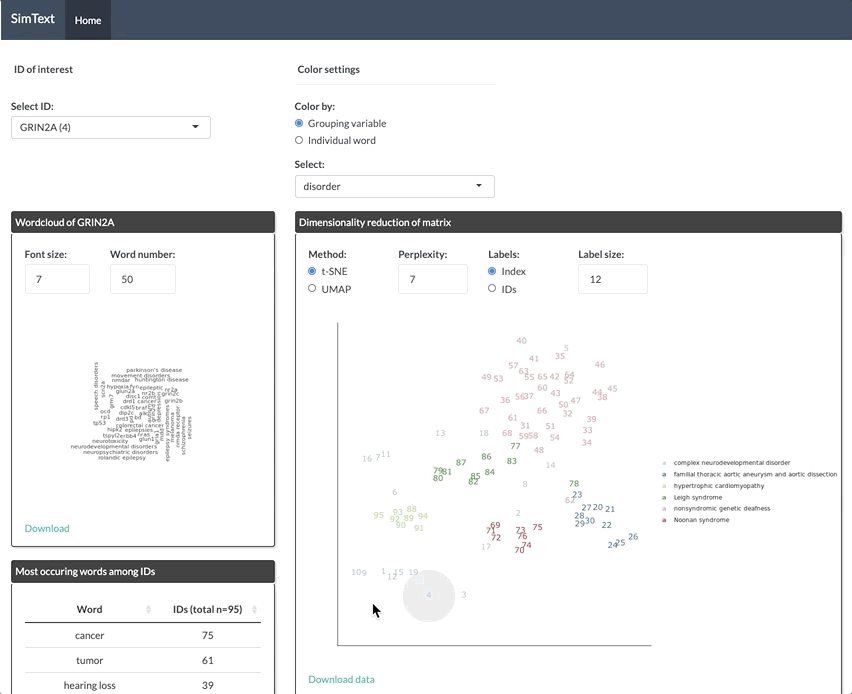
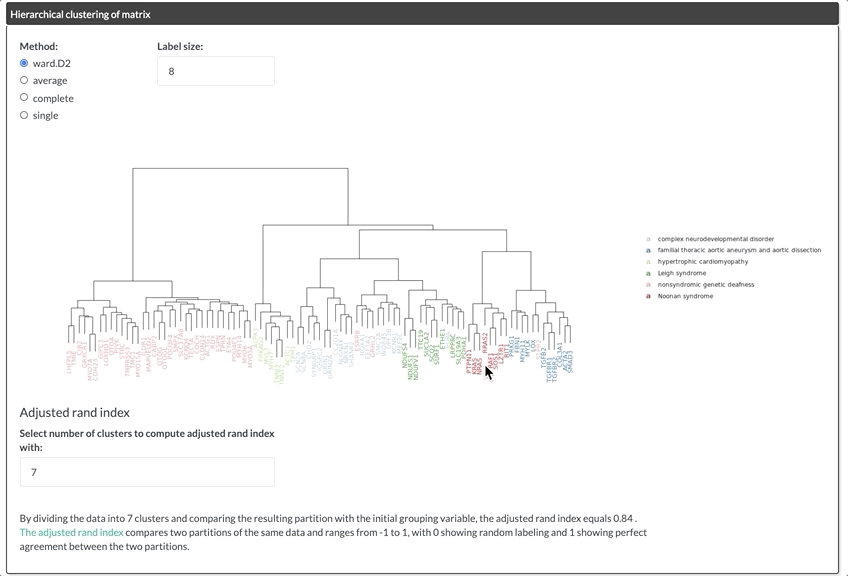